The Longevity & Aging Series is a collaboration brought to you by Aging (Aging-US) and FOXO Technologies. This limited series of video interviews invited Aging researchers to speak with researcher and host Dr. Brian Chen. Dr. Chen is an adjunct faculty member at the University of California San Diego.
—
Below is a transcription of the first episode of the Longevity & Aging Series, where Drs. Alex Zhavoronkov and Frank Pun discuss, in detail, their recently published research paper, “Hallmarks of aging-based dual-purpose disease and age-associated targets predicted using PandaOmics AI-powered discovery engine.”
Okay, welcome. This is the first of a long series. I’m Brian Chen, Chief Science Officer of FOXO Technologies. With me today are Doctors Frank Pun and Alex Zhavoronkov. They’ve recently published a paper titled, Hallmarks of Aging-based Dual-purpose Disease and Age-Associated Targets Predicted Using PandaOmics, which they’ll tell us about, which is an AI powered discovery engine.
So before we get into the details, why don’t Alex and Frank, you guys give us a brief introduction about yourself and your background. Maybe we start with Alex.
Sure. Hi, happy to be here. My name is Alex Zhavoronkov. I am the founder and CEO of Insilico Medicine. I’m also the founder and now independent consultant, Chief Longevity Officer of a company called Deep Longevity. My background was in computer science. I did my first two degrees at Queens University in Canada. So I’m Canadian. And then had a really fun career in information technology, primarily in semiconductors, in GPUs that are now powering deep neural networks.
And in early 2000s, I decided that I wanted to switch from IT into biotechnology and dedicate the rest of my life to the study of aging. I did my grad work at Johns Hopkins University, I did my grad work at MSU. And then worked for a number of companies in the field, I had my own lab at the Cancer Center, regenerative medicine lab and bioformatics lab.
And then in 2013/2014 started Insilico Medicine. Originally, went back to Baltimore to the campus of the Johns Hopkins University, Emerging Technology Centers, started the company there, but it was already very truly global from scratch. So now, most of what I do is manage a very global team distributed over eight countries and regions. We have six physical R&D Centers, and we have over 200 employees and over 200 FTEs.
So now my background shifted more into AI-powered drug discovery, and very much everything I do is outlined here on those stages. So we do Target ID and Validation, Hit ID, Lead ID, IND-Enabling Studies. We are now in clinic with the first AI discovered and AI designed molecules. So my background is transforming and transitioning more into pharma and AI powered biotech.
Dr. Brian Chen:
Great. I’ll just make note that I also spent some time in Baltimore and your legend was already growing by that time, maybe over six years ago now. So you’ve built quite the establishments here. Great to have you here.
You’re accompanied by Dr. Frank Pun, also at Insilico Medicine. So Frank, would you like to introduce yourself to the audience?
Yeah, sure. Thank you, Brian. First of all, I’m really happy to be here. Well, I’m currently leading a team of application scientists focusing on our target discovery platform, PandaOmics. So my goal is to, with the latest AI technologies to help the medical scientists to find the best therapeutic targets.
My background, I got a PhD in Biochemistry and then upon my graduation, I was working as a resident scholar and leading a team of scientists focusing on the research on some neuro diseases studies and cancers. And before I joined Insilico, I served as a CEO of a biotech company in Hong Kong, known as PharmacoGenetics Limited, focusing on some diagnostic products environments, as well as the sequencing.
I joined Insilico in 2020. It is a very good opportunity for me to explore the latest advances technologies in Insilico. Actually, I also got a MBA from the Rutgers Business School in the United States. Yeah. So currently in addition to leading the applications team on the target discovery, I am also responsible for a lot of project collaborations in both academia, as well as the pharma. Yeah. That’s my main background. Thank you very much.
And I’m really happy to have the opportunities to introduce our platform as well as our aging paper today. Yeah. Thank you.
Dr. Brian Chen:
Perfect. Well, great to have you here. Just because our audience at Aging is very broad so we have laboratory scientists and we have computational folks. You guys are certainly on the extreme end of the computational side, but you have a lot of biology in your paper so you span both. Can one of you give a brief intro for those who’ve never heard of AI, what it is and why one might use it? And then let’s talk about PandaOmics and what that specifically does?
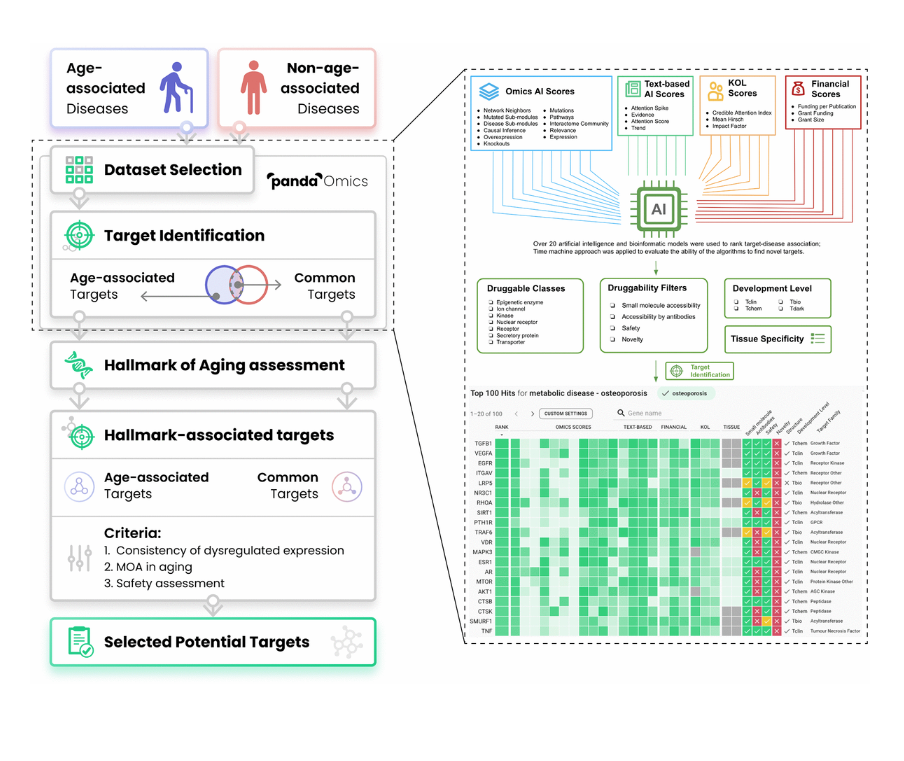
Dr. Alex Zhavoronkov:
Yeah, this is a very interesting question. There are multiple different definitions of artificial intelligence and different scientists define it differently. So for me, the way I look at artificial intelligence is I associate it with deep learning and deep reinforcement learning. It’s a form of machine learning, which mimics the human brain. So you’ve got multiple layers of interconnected neurons that changed their connections and their different properties with exposure to data in very different settings with different algorithm approaches.
So those deep neural networks, there are multiple flavors of deep learning. There is deep reinforcement learning where you combine prediction recognition generation with strategy so now deep neural networks can learn strategy. And those combinations allow you to outperform humans in strategy associated problems like games of Go, Atari and many other tasks, including tasks where the strategy is not very clear.
And I’ll just share my screen very briefly. A picture is worth 1000 words. Here on this slide you can see the history of deep learning. Some of the very early concepts were proposed by Alan Turing and many other brilliant minds at the dawn of the 20th century. Many of the technologies had to be developed and converge before 2013, 2014, when there was a real explosion of AI when deep neural networks started outperforming humans.
And the reason why AI became so popular and trendy around the time is because three things happened, first, the algorithms became better and caught up with the availability of data. So now those deep neural networks could be now could be trained on massive datasets that became available. Of course, that’s the other big thing that allowed the deep neural networks to start to outperform humans.
And third is GPU computing. So the availability of highly parallel, high performance computing graphics processing units, those semiconductors that can outperform many parallel operations at the same time and are used to power video games, the performance there has improved to the level where again, deep neural networks could be trained very efficiently, very quickly on large amounts of data. And those deep neural networks started outperforming humans.
Then there were several innovations that transformed the industry. So one is Generative Adversarial Networks. That’s a form of AI imagination. So GANs, those are two deep neural networks competing against each other. One is learning to generate meaningful noise with the desired properties. And another deep neural network is trying to recognize whether the output of this first deep neural network is true or false.
And those two deep neural networks compete with each other over millions or billions of iterations. They get so good at generation of fake data with the desired properties that it’s indistinguishable from reality. So now you can generate really efficient, really beautiful faces of humans. Really great works of art. You can now generate the molecules. You can generate human biological data. You can actually, so we use it a lot for generation of virtual populations.
So now you can take, imagine one human with all the properties, imagine that you take Brad Pitt and you want to make Brad Pitt older. Let’s say 80 year old female Asian and a different skin color. So those are adversarial generation conditions. You can tweak them and get the entire distribution you can get hundreds of thousands of Brad Pitts where those four conditions are satisfied. And you can do this on biological data, or on data that is are not pictures. You can age humans including into the future.
There is also great technology called reinforcement learning, which basically works on reward and punishment concept. If the system produces the results you want and identifies the strategy, it gets rewarded. If it doesn’t, it gets punished. And there are many approaches to this concept, but now those systems are outperforming humans in strategy games, including Atari, and even extremely complex ones like StarCraft. And the company called DeepMind, truly pioneered that technology. It can also be combined with GANs. So think about those many technologies as Lego blocks that you can build very sophisticated AI systems.
And finally, another really important technology that just emerged are transformer neural networks. These networks are predominantly used on text data, but many other data types can be used on transformers. And transformers allow you to get very important insights from data.
So for example, you’ve probably heard about GPT-3. This is a form of conversational AI. So you can even create very efficient chat bots that will mimic human behavior and human responses providing very efficient job ready type answers.
So here on this diagram, you can also see the timeline for Generate Adversarial Networks. Here are just general purpose GANs that can generate again, those deep fakes. And here are the GANs for chemistry. And to my knowledge, the first paper on GANs for chemistry in a peer review journal was our group paper, A Cornucopia of Meaningful Leads. So there we, for the first time show that we can use the Generative Adversarial Autoencoders to generate molecules with the desired properties and then validate it by using matching to the non chemical space. And then you can see that there are theoretical papers that are group published, different approaches, different Generative Adversarial Network architectures applied to different chemical problems.
Then in 2017, we did our first experimental validation of a newly generated small molecule with desired properties, validated, tested, published, and we got our first round of investment, the first series A investment. And then we also did another famous paper where we generated small molecules with the desired properties in a very short amount of time, synthesized and tested. And that is a pretty famous paper. So that’s what AI can do for you in chemistry.
And today we’re going to talk about what AI can do for biology, because when you are thinking about drug discovery, you need to think end-to-end, and there you need to have multiple forms of AI applied to different types of problems.
So actually, if I can very quickly show you how it looks like from the integration perspective. So if you want to apply AI in drug discovery, you need to look at the mini steps of drug discovery and development, look at how you can automate and accelerate and improve every single step and then connect those steps with AI.
And you would be using very different types of AI for every one of those steps. So think of it as a brain, right? So you’ve got pieces of the brain that are responsible for vestibular apparatus so you do not fall. You have memory, you have higher cognitive functions, you dream, or you strategize. So those are different parts of your brain. Very seamlessly integrated.
So here, we’re trying to build the AI pharma brain. And for that, you need to have AI for clinical data analysis. You need to have AI for competitive landscape analysis. For transcriptomic and proteomics data analysis. That’s what we’re going to talk about today. Drug effects, research, structural biology, but many areas of drug discovery cannot be significantly accelerated by AI because you need to do the experiments. You need to do physical work. So that’s going to be AI for drug discovery the way I see it. And what we have done we have integrated many of those steps.
Dr. Brian Chen:
Great. Yeah. Just to set the stage for us, PandaOmics and then what you just showed us, how are they related?
Dr. Alex Zhavoronkov:
Sure. Again, the picture is worth 1000 words. So PandaOmics is the first step. It’s the step where we identify targets. We do biological data analysis and identify targets for a variety of diseases. Let me just show you one slide.
So PandaOmics is a tool that stands in the very beginning of the drug discovery process. So you can use multiple different data types, predominantly transcriptomics, proteomics, methylation, and text in order to identify targets that are at the same time novel and have high confidence of working in a specific disease or in a specific biological process like aging.
So here you’ve got 60,000 possible targets or more than 10,000 diseases. And what we’re trying to do is match the target to a disease. So you would be able to modulate the disease or a biological process. Chemistry42 system allows you to very efficiently generate small molecules to achieve that goal, to basically be able to manipulate those proteins with chemistry. InClinico predicts the outcomes of clinical trials and improves your ability to design clinical trials.
So if you look at it from a broad perspective, Frank is going to talk about that. We start from target discovery usually, and that process is more of an art than science. So it’s art and science, because at the end of the day, you will have a very large list of promising targets with the desired properties and you would need to rank those targets because you don’t have an unlimited budget. And very often you just need to select one.
So one of the highlights of what we do at Insilico was our exercise that we started in the end of 2019, beginning of 2020, where we decided to go after a novel target, novel molecule, generate using AI and take it all the way into clinic. So right now that particular target is in a clinic. It’s a very improbable event. Usually the probability of that happening is very tiny. It’s less than a percent because most of the targets fail at the early preclinical stage, but in our case, we managed to succeed. And that is due to the system that we’re going to show you. So we try to do the same thing for aging and age-associated diseases in this paper.
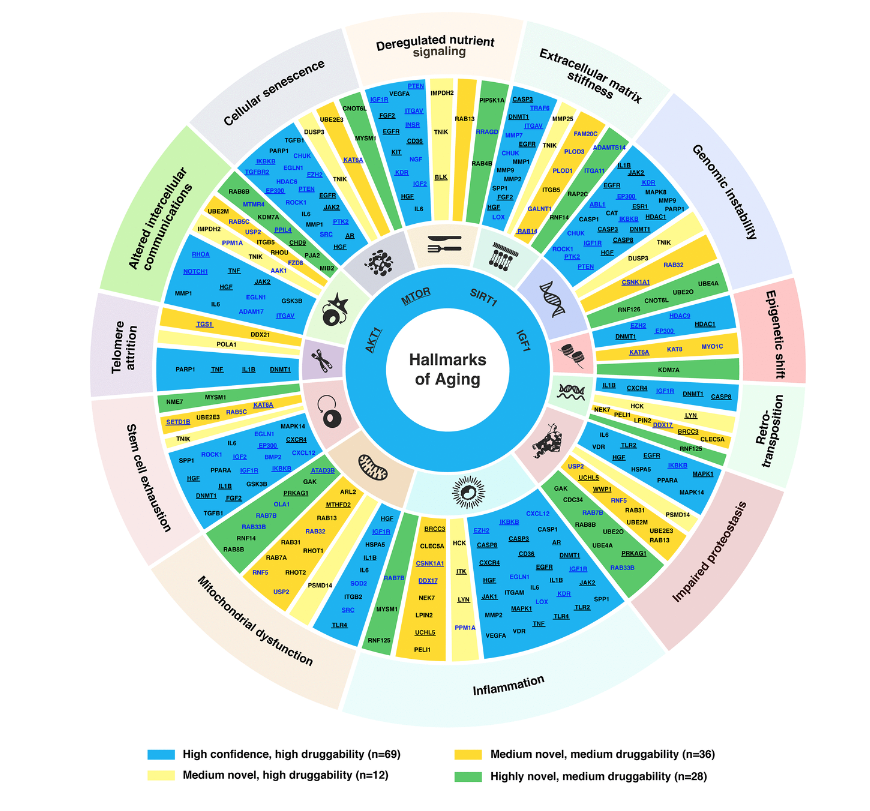
Dr. Brian Chen:
Frank, so now we could get into your paper, which as Alex just set us up for is focused on aging and age related diseases.
Dr. Frank Pun:
Yeah, sure. Okay. But before that, maybe I can give more background of our PandaOmics platform. Let me share my screen now. Good. So as Alex mentioned, PandaOmics is the first step of our fully integrated drug discovery platform. And mainly focused on the target discovery as well as some analysis.
Previously we have demonstrated very a successful story that is we use our PandaOmics to identify a novel target and then use Chemistry42 to find that the design of molecule. And then went through a series of in vitro and in vitro studies and reached IND-enabling enabling in 18 months. And now we already reached the phase 1 stage.
So basically AI can shorten the time and the early stage of the target discovery as well as the lead automatization. So from this AI can speed up the drug discovery and development significancy.
Let’s focus on PandaOmics. As I mentioned, it is an end-to-end analysis as well as the target discovery platform. So in our back end we have huge resources. For example, we have over 10 million samples from different types of the datasets, including, but not limited to microarray, RNA-seq, methylomics and proteomics and the single cell data. And then for the text data, we cover not only the publications, but also the grants, patents and clinical trials, as well as many financial reports.
So here is the overall workflow of our PandaOmics. Basically we can start with any in our platform or we can upload our proprietary datasets into the platforms to do the analysis. And then we can start with the standard gene differential expression analysis, pathway analysis. And then based on these, we can find out the gene that is highly associated with the disease, and then with a lot of the drug ability filters, and then we can find the targets we want. So this is the basic idea.
So in terms of we have developed our proprietary tools known as the iPanda evolutions. And in 2016, we already published a paper in Nature Communications that demonstrated our pathway analysis, outperforming all other pathway analysis tools. And over the years, we have published many papers with these iPanda tools. And in PandaOmics, we already incorporated with the latest version of this iPanda Pathway Analysis.
So here is the overview of our target identification philosophy. So in PandaOmics, we incorporated a lot of the omics AI scores, text-based AI scores, KOL scores, and financial scores. So all together, we have over 20 AI and bioinformatics models where we use it to render target disease associations.
And then we also use the chemistry approach to evaluate the abilities of our evolutions to find the novel targets. And then after that, we have a series of druggability filters, for example, to further narrow down the druggable class, we are interested in and then also assess the drug ability of the targets, whether they can be accessible by the small molecules or proteins, the target safety and the target novelty, which is very important to the researchers.
And then we also provide the filters for different developmental level, yes, so that difference users can… So this is our main philosophy.
So I guess many people now will ask, so where is the AI components of the PandaOmics? So basically AI is used to identify the genes, diseases compounds for those biologic processes and the associations in the text with our NLP. Okay. The most important is AI driven graph-based model is used for ranking the targets. Yes. Which I will give more detail in the next slide for the target identification.
And lastly but it’s also very important that is AI is also used to capture the trends to analyze the potential for the initiation of phase one.
So as I mentioned, we have over 60 models to help us to run a target disease associations. And we finally only selected about 20 models into our platform to do that. So I would like to take the interactome community as an example here. For example, we will collect all mixed information, text data of our genes, and then put them together with the disease information, into a single bipartite graph. And then we will see how well the genes is being connected with that particular disease.
And then we will do the target disease pair embedding, and then we use multilayer perception decoder, to get a prediction score for how well that gene is being connected with the disease. So it is only one of the models to help us to predict targets and disease associations.
And then all these over 60 models, we will try to validate where our model can really help us to predict the good targets. Then we use a time machine validation approach. Here for example, we take 2010 as an example here as a cut off time. And then our system, we try to collect all the data between before 2010 and use our model to protect the targets coming out into the clinical phase in the next several years.
For example, let’s say if the model can predict [inaudible] target that will come into the clinical trial in the next several years, it means that the model performs very well, but let’s say it only one or two. Okay, in that case, we will further to improve our algorithms or we simply ignore that model. So this is the time machine validation approach.
In that case, over 60 models, we will have their validation matrix. The X axis shows the novel change enrichment of how well the target predictions that model can do. And then the Y axis shows whether that target prediction enrichment is statistically significant. Yes.
Yes. So here we can just clearly see that Score 2 has a higher predictive target predictive power than Score 1. In that case, we will only choose Score 2 as the model to help us to predict on targets in our platform. So this is our validation approach.
So basically I think our PandaOmic’s unique advantage over the other tools in the market is that we have a huge database that is created specifically for the target discovery. Yes. And we have a very comprehensive source of data. It is not only limited to omics, but also the text data as well. And also we have a very unique time machine validation approach because we all know that we cannot predict the future, that with the back testing, we can still prove the abilities of our models to generate digitally low target hypothesis.
And other points that I would like to highlight is that most of the AI and statistical testing models to calculate the targetID scores in real time. So it is actually a dynamic calculation of the targetID scores will vary depending on the inputs of the datasets the users add into our platform. So it is not just an encyclopedia or just a database telling you what target is associated with what disease but really based on the input data of the user, into our platform.
As we know that in Insilico we have one of our main focus is on aging in previous year, we published the main paper on that, for example, of our markers of aging, some aging clock as well as some different examples. For example, one of the latest paper is the Increased Pace of Aging in Co-related Mortality. Something like that.
So in that case currently, we use these grade platforms to help us to find some dual-purpose targets. I would like to explain why do we need to find the dual-purpose targets? Because nowadays most of us agree that aging is one of the very important driving force for many types of disease. So in that case, let’s say if we have a dual-purpose target that is implicated in both age-associated diseases and the aging, even the target itself cannot be very helpful for specific disease indications, but the people, the patients can still get benefits from the targets itself. So it is known as the off targeted effect. So it is very important. That’s why we conducted this study.
Here is the main workflow of our present study. So basically we tried to identify a series of age-associated diseases, and then many non-age-associated diseases. And then we gather the corresponding transcriptomic data and some mix data in the PandaOmics. And then we do the target identifications. Here is the target identifications. I can show more details with our platforms to how we can do that.
And then we can find age-associated targets here. And there are some non-age-associated targets here. So the overlapping, we will count it as the common target. And then we will do the common aging assessments to make sure the target we select will be involved in aging related pathway or some MOA. And then after that, we will identify the age-associated target and the common targets.
And then next, we go through a series of criteria, for example, whether those targets are being consistently dysregulated in a couple of diseases, that is very important. Yes. And also their MOA in aging and also the targets safety. Yeah, because some targets may be very good, but if it is not safe to use it and then we will not propose that. And finally, we select some potential target for this dual purpose.
In terms of hallmarks of aging we have basically a connected trail of main mechanisms, for example, some of them are the most important ones and such as the inflammations and also the Telomere attrition. We have to note that the extracellular matrix stiffness is also very important and it is one of the important hallmarks of aging proposed recently. And our paper also demonstrated it is very important because just over 30 of our targets selected belong to this family.
In terms of disease, we selected 19 non-age-associated diseases and 14 age-associated diseases. The main criteria is that let’s say if the onsite age is highly related to the particular indication, we will classify that as an age-associated disease. But here we will simply exclude, let’s say the cancer and some cardiovascular disease for this study, because we think that, especially for the cancer, some of the mechanisms of the hallmarks of aging cancers and the age-associated disease are in the opposite directions. So in that case, we simply exclude cancer here for the present study.
So I hope you can see my screen now as well. So here is our PandaOmics platform. So basically now I’m showing the target identification results of one of the age-associated disease. This is ALS, which is also one of our next focuses. Most likely we will publish other paper on this ALS as well. So here we can see that there are over 20 omics and text based models for the type of discovery. So this is of our ranking, shows us some of the potential targets for this ALS. And now here shows the 13 omics model here.
So each of them has passed through the time machine validation approach and show that they perform very well in terms of target identifications. That’s why we include them here. So what I would want to highlight here is that these omics scores models they are based on the real time calculations depends on dataset we input into the system. On the other hand, the text based scores, they are pre calculated. They’re relatively static. Yes.
So by default, let’s say when we find high confidence targets, we can turn on all this omics score model, as well as the text based model so that we can find the target that have a strong performance in terms of the omics model as well, have a strong backup from the literature. That’s what we do.
At the same time, let’s say if we find high confident target, we can make sure the target has already has been associated with some small molecules and it is relatively safe to use and then we make sure the target belongs to a druggable target. So that is how we find the high confidence target.
So next let’s say, if we want to find some novel target or some media novel targets, how we can do that. Okay. This is very simple. For example, in that case, we can relax the small molecule requirement. In that case, we can find a target that currently do not have a small molecule associated with them entered in the clinical phase, that they still belong to a druggable class. That is very important because we don’t want to find something that belongs to a non druggable class. Then it becomes very useful for us.
And then we can turn on the novel filters to make sure they are relative new, and then still turn on the druggability filters. But now, because we want to find some novel target, we do not rely on the literature support. So now we can only use the omics models to find the targets. So in that case, and the system will based on our new criteria and then to propose a list of feasible targets.
And for example, here, these targets, they are very new to this particular disease. As we can see, we didn’t get any leader support from that text database, but why did the system select them? Because these targets still perform very well in their omega model. At the same time they fulfill our druggability requirement. For example, these targets can be accessible by the small molecules, then they already have some such known structure. And then they belong to a druggable family. That is very important.
At the same time we can further to narrow down or to run these target based on our preference. For example, let’s see if I think that expression is the most important. And then I can sort by the expressions. Or if I think the interacting community or even the pathway is relatively more important and then I can sort by the pathway. So it is a very flexible tool. So this is the approach, how we can find the high confidence target as well as the novel targets for a couple of age-associated disease and those non-age-associated diseases.
So now I will come back to a slide representation. So after we use the PandaOmics platform identified the top 100 targets for each of the disease. And then we gathered high convinced targets with different novelty, for example, high confidence medium novelty, and highly novel targets. And then we just prioritize them based on their occurrence, as well as the average ranking across multiple diseases. And then we gather the top 100 for each of the novelty targets.
And then we do the hallmark assessment to find the high confidence medium, and novelty targets. And then we allocate them into our target based on different hallmarks of aging. At the same time, we also need to do the expression analysis to make sure the target, we selected they have a consistent dysregulation directions in a couple of diseases. And finally we select the target.
Here is the examples of the high confidence targets we find in the 14 age-associated diseases. As we can see some of the targets, they are commonly chosen by the system, for example, this CASP3, VEGFA, something like that they appear in all the age-associated disease targets.
And then here, we just give very detailed information for the target’s performance, according to each of the diseases. And also we separate them into two different disease classes, for example, the neurological, metabolic, inflammatory and also fibrotic disease classes.
Okay. So from here, we can see how well the targets in each of the disease areas. And then now we just do a VEN diagram and then we can find which target is specific to the age-associated disease. And some are for the non-age-associated disease and some common targets. Sorry.
And then we apply the same for the medium novel targets as well as the highly novel targets. And finally, we gather a list of 145 targets, that is of a very good performance overall. And then we allocate them into this target wheel. So a very important figure in our paper. Yes. Because it summarizes the high confidence target, as well as the medium and highly novel targets based on their druggability as well.
So from here, let’s say if I want to develop some drugs for focus on repurposing, and then I can choose the high confidence targets. For example, let’s say we can find something that is, let’s say, choose a inhibitor to restore the targets dysregulated expression patterns in a particular disease. And then for the novel targets, it is very good to do the, let’s say the validation studies and then to design a new molecule for that path to speed up the drug development. So this is the main idea.
And also from here, we can see that there are some targets involved in many of the hallmarks of aging, for example, IGF1, and also the AKT1, they are in most of aging. So it is probably due to their involvement in lots of the aging or non-aging pathways. And so far we find that information is the most important factor of the hallmarks of aging, because it has the highest number of the aging target we selected.
And then next, we also do the expression and analysis of all the targets we find in 40 different age-associated disease targets, because we want to find some target that is the consistent dysregulation in one direction, in most of the disease cases. Based on that, we can further to do the pathway enrichment analysis with all those 145 AI-derived targets. And then we find that they are relatively found in some of the very well known aging pathways. For example, the FOXO PI3-AKT and also the MAPK centering pathway.
And finally we map these targets, the target we find into these age-associated signaling pathway here. Yes. As we can see, PandaOmics can find many of them.
And lastly, based on the Hallmark of Aging Assessment and Expression Analysis, as well as the Safety Assessment, we finally propose a list of the potential dual-purpose targets that is in this table.
Okay. Altogether we propose the nine targets with their upregulated or downregulated in each of the disease classes, with a very detailed role in aging. And of more importance as we can see from this clinical trial status, okay, I think for example, high confidence targets, most of them has been completed in phase 4 and most of phase 3. For these targets, maybe we can use it as a drug repurposing, but at the same time, as we can see, there are many targets that do not have any clinical trials reported. So they are relatively novel. Yeah. So we can do further experiments to validate them and then to speed up the drug development process. This is the main idea.
And then finally we suggest for genes across different disease classes. The reason we select them because they have a significant difference in all of the disease expression comparisons. And also there is a significant difference between their expression for all those age-associated disease and non-age-associated disease. At the same time they fulfill our druggability requirement so that we think they are very good candidates.
So I think this is the main idea of our paper. So yeah, I think that’s it. So hopefully we hope this approach can really speed up our research in aging and finding the dual-purpose targets can really benefit not only the people who have a particular disease, but the overall aging population as well. Thank you.
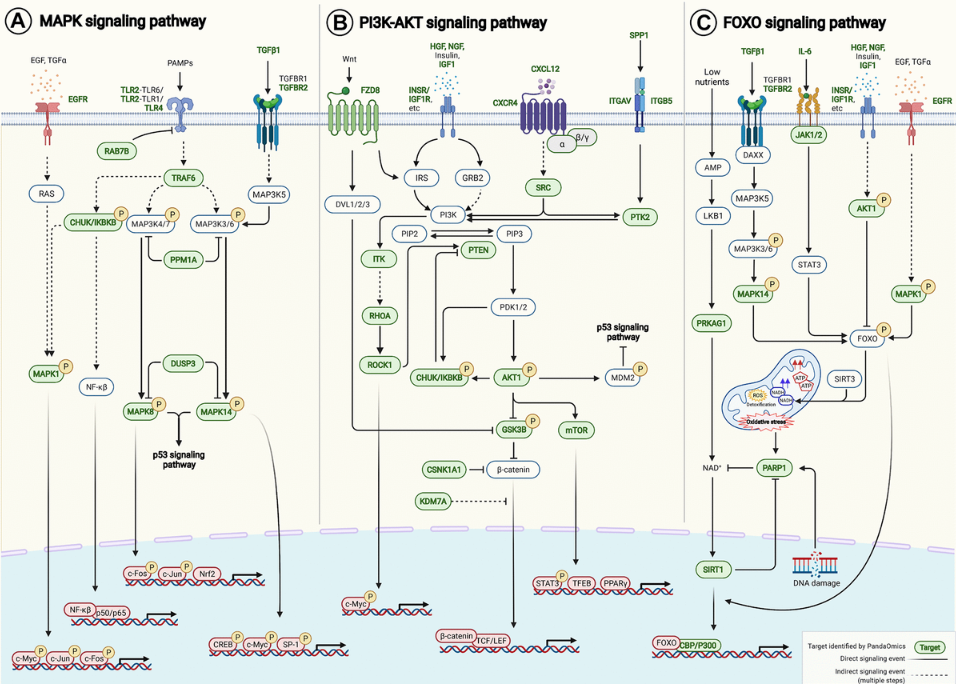
Dr. Brian Chen:
What could bring this tool to the next level?
Dr. Alex Zhavoronkov:
Well, if you are talking about the future of this paper, the most important aspect is of course experimental validation in humans. And the way to approach it is to test those targets in certain age-associated disease models. So with this paper, we provide a pathway for possible clinical development of interventions that are implicated in age-associated diseases and aging itself. Right?
So for aging, as you know, it’s very difficult to test or develop targets in a way that they make them commercially tractable. And you could possibly take some of those targets, test them in age-associated disease models, to see if they work, if they do, if you take them further, you could possibly repurpose them later on for aging as well.
I don’t think that significantly extending lifespans of animals using drugs that work on those targets would provide a huge proof of concept for the clinical development in humans for aging, as animals do not age in the same way we do. So there are of course, many, many common mechanisms, but it is just that they die of usually different causes. And we don’t have the same diseases.
So you don’t see a lot of mice developing Alzheimer’s or Parkinson’s or other diseases in that age-associated disease category. They just don’t live so long. Don’t accumulate the damage in the same way. Don’t cope with the damage in the same way. So that is why I think it’s extremely important to focus on those longevity therapeutics that are commercially tractable that have the potential to work on a human disease. And once they are in the market, you would be able to try them for aging also in humans, because the best model for human aging is a human.
And I think that right now, we need to learn from some of the drugs that are out there, like metformin and rapamycin and a few others that are implicated in age-associated diseases, that are implicated in aging. But since those drugs have been on the market for a very long time, they’re not exactly commercially tractable right now. So nobody would want to develop rapamycin for an age-associated disease, because it’s an old drug, the patent life is not there anymore. And it’s very well known.
So for repurposing, what we provide here is potential novelty. So you spend five, 10 years developing and marketing, and then you still have another 10 years of patent life, patent protection that will enable you to recover the costs if you are successful.
So yes, it’s basically, we’re talking about 10, 20 year horizons, but I hope that some of those targets will be both commercially tractable and useful in aging. Just like rapamycin, and imagine that if you have commercial rights to rapamycin while it’s still on patent, you of course can purpose it towards multiple diseases. You can fundraise for that. You can do more trials, you could start selling that drug in a variety of diseases. You will start getting biomarker data from real patients. You would be able to implicate it in a disease. Currently it’s very difficult because again, it’s off patent.
So those aging targets provide you with a pathway for possible clinical development and commercialization of those drugs in aging and age-associated disease. But again, the next step would be to test them in animal models and establish the body of evidence that the system that we’ve developed can hunt for promising targets in age-associated diseases. And here again, we’ve implicated them in aging as well, and structured them by hallmarks of aging so we hope that will help the community to develop the promising field of longevity pharmacology.
Many of those targets might be able to be purposed in a way that it would be picked up by a biomarker by a aging biomarker. Of course not a epigenetic biomarker because a epigenetic biomarker probably would be good for epigenetic drug bot or epigenetic targeting drugs. For some of the more precise, broader biomarkers, you would be able to see the difference between before and after treatment. So we are also working on an additional tool that will allow you to develop biomarkers that will pick up the effects of those drugs that are targeting those promising targets.
And finally, I think that right now we’re just scratching the surface of longevity pharmacology. Because even if you look at rapamycin today, it’s actually, you don’t know how to take it. We don’t know how to properly administer it for aging or for age-associated diseases. Do you take it once a day? Do you take it once a week? Which dose do you want to take? What kind of effects are you achieving when you are taking five days a week, once a week? What kind of effects are you achieving if you are taking it five mg a day, how does it affect the overall body function? Are you getting any benefit? Are getting worse? Are getting any efficacy?
So even though that’s one of the most popular drugs in oncology and in several other diseases in organ transplantation, it has 20 years of history we still don’t understand how to use it. So it shows you the complexity of pharmacology in general, those targets that we’ve published is just the first step in a very long journey. Because even if you implicate them in a disease, even if you implicate them in aging, you then will need to still have a protocol for administration and tracking and that’s going to be difficult. That’s going to be a big journey.
Dr. Brian Chen:
That makes sense. Obviously, identifying drugs and getting them to market is an immense task. Did you guys find in this current paper that the data sources were adequate enough?
Dr. Alex Zhavoronkov:
Well, to answer this question, you need to look at the different data types that were used for this study. So of course we used publicly available data. Here we also used models that were trained on massive amounts of publicly available, also private data. So the models there are trained on massive amounts of data that very often is not available for public consumption. And many of those models would perform well on data that is reasonably noisy. So even if you have a lot of inconsistencies, like some samples are bad, the system will facilitate for that. So you would still be able to get adequate results.
In some cases, of course, if the entire dataset is crap, you are not going to get great targets. And for that, we do have quality control systems that enable you to evaluate the quality of the data. We even have the scores that ranked the scientists associated with the study and the trustworthiness of this data.
And finally, since to nominate a target for this list that we’ve published, you need to have many different pockets of evidence to align. So the transcriptomic scores need to tell you that it’s a promising target implicated in this disease. There needs to be text based scores, commercial tractability scores, druggability scores. When we are talking about omics approaches, there are many, many different scores. So when they align, it gives you much more confidence that the target is real.
And of course, some of those targets may have challenges, and you would see that. And some of those that would come from that bad quality data, but usually when you’re taking 10 or 15, it’s not that many for validation. So you can actually use already existing chemical matter to very cheaply test those. Or reasonably cheaply start the drug discovery process to get tool compounds, some very promising hits to be able to validate those targets.
Usually in a program in the drug discovery program, you are not starting with a single target. You’re starting with a list. And the failure rate there is 95% anyway. So here you should have in theory, higher probability of success at the target discovery stage.
Dr. Brian Chen:
That makes sense. And I think Frank, you showed some in one of your results graphics that you guys were able to recapitulate using your data, your approach, some of known aging targets or systems that we’ve already known about, inflammation, rapamycin I think. Yeah. mTOR was there for sure. Yeah. Yeah. I think ultimately the proof is in the pudding, and if you’re able to recapitulate those things, that’s all that matters. Right?
One other question about just the approach. I think on this paper in particular, you focused on aging and age related diseases, but I suppose that the concept, the tool of PandaOmics itself can still be used for cancer drug discovery or any other disease specific discovery. That’s correct?
Dr. Alex Zhavoronkov:
That’s correct. So most of the partners that we work with, so right now PandaOmics is actually a very broadly used platform. So a lot of people are using it, including academics, mostly smaller biotechnology companies that are still doing research. Of course, a lot of big pharmaceutical companies. So I would say 80% of these take the cancer samples, I can take the norms and very quickly do the norm to disease analysis and you don’t need to be working with the tissues that are very remote from where the disease happens, or it’s usually not a systemic effect that you’re looking at.
For many diseases you really need to first formulate the hypothesis of where it’s coming from and then start doing the analysis. PandaOmics allows you to also work with text data quite efficiently, right? So it incorporates a lot of different text datasets and also provides you with additional hypotheses coming from those non-trivial hypothesis coming from those text datasets. So even if your omics dataset is wrong, the text based scores can still pull out good targets.
Dr. Brian Chen:
Here’s a fun question. Why the name PandaOmics?
Dr. Alex Zhavoronkov:
Well, we have an algorithm that we’ve developed a long, long time ago. It’s called iPanda. So it’s a Insilico pathway activation network decomposition algorithm. And it just stuck. And I just like the animal a lot. So it’s one of those animals that is super benign and never harmful. So we like panda and just extended the algorithm name to the product name.
Dr. Brian Chen:
Perfect. Love it. Both of you are in a unique position. So I want to ask this question. Certainly other industry groups don’t really publish that much or are a little bit more secretive. In this paper I saw the main targets listed and with extensive description. And I think I’m just curious if there’s a message that you could send to other companies assuring them, that they’re not going to lose their IP, what steps can they take to be good stewards within the scientific community, but still be profitable, et cetera?
Dr. Alex Zhavoronkov:
Sure. So I think that it’s extremely important to publish, because you’ve seen that companies that are secretive are very often associated with bad stories. You’ve seen those stories with Theranos. You’ve seen those stories with many others. You actually have seen those stories, even with some of the very promising, successful longevity companies. That if they had a little bit more peer review and transparency other people could step in and help them correct their path so they would not fail and would not impact the industry so badly.
So what we started doing from the very beginning is that we came from academia, and we decided that we will publish whatever is possible in the most transparent way possible. And it actually helped. So investors, for example, when you are talking to them, if you want to deliver a point across and they have questions, and usually they bring key opinion leaders, you can give them a paper. And if they are questioning target discovery capabilities, you can give them a paper or 50 papers saying that, “Okay, well here is how it evolved. Here is the thinking. Here is what worked and here is what didn’t work.”
And they actually like it because at the end of the day expert investors, they’re also academics, so they also have some academic background. I think that it’s extremely important for companies to publish because those publications, they help even investors and regulators to trace your progress. Because those companies that all operate in this field, they very often play a very long game. So we are here for a couple decades or longer. And very often you have to pivot. Very often, you have to rest or put the pedal to the metal. And on some programs you need to accelerate or choose some of the more promising programs. And those publications they remain. And if you pivot, people can still learn and understand whether they should go into this or not. So you are also providing a service to a community.
So I think that transparency is important. It’s important to have a very collaborative network of partners that also like to publish and ensure that academics are always involved. Because very often what I see in our field in AI power drug discovery, or in AI in general, innovation is actually not driven by the academics. So you see that some of the academic institutions in some areas are like 2, 3, 4 years behind. So it’s just not possible for them to have such a sophisticated purpose built team that is not out there to do a paper. It’s out there to really solve a problem, because that’s what you need to do when you are a commercial company, you need to be able to sell and solve.
And very often the innovation that happens when you try to solve a problem for real, it’s not going to be visible if the company doesn’t publish. So it’s important to publish the proof of concept so that even the academics could get a little bit better line of sight into where to put their efforts.
Dr. Brian Chen:
Very nice to talk to you, as always very inspiring. We appreciate your time. We already lost Frank Pun. So thank him for his time as well. So until next time, good luck with everything.
Dr. Alex Zhavoronkov:
Perfect. Thanks so much. Great that Aging started doing those video series. It’s very nice.
Click here to read the full study published by Aging (Aging-US).
AGING (AGING-US) VIDEOS: YouTube | LabTube | Aging-US.com
—
Aging (Aging-US) is an open-access journal that publishes research papers bi-monthly in all fields of aging research and other topics. These papers are available to read at no cost to readers on Aging-us.com. Open-access journals offer information that has the potential to benefit our societies from the inside out and may be shared with friends, neighbors, colleagues, and other researchers, far and wide.
For media inquiries, please contact [email protected].