Dr. Liang-Kung Chen details a research paper he co-authored that was published by Aging (Aging-US) in Volume 14, Issue 3, entitled, “Predicting neuropsychiatric symptoms of persons with dementia in a day care center using a facial expression recognition system.”
Behind the Study is a series of transcribed videos from researchers elaborating on their recent studies published by Aging (Aging-US). Visit the Aging (Aging-US) YouTube channel for more insights from outstanding authors.
—
Hi, this is Dr. Liang-Kung Chen from Taiwan. I’m a professor (at the) Center for Health and Longevity and Aging Sciences of National Yang Ming Chiao Tung University, Taiwan. I’m also a clinical geriatrician working in the Taipei Veterans General Hospital.
Today, we’re glad to share our recent work that is published in Aging. And the topic is predicting the neuropsychiatrist symptoms of older people with dementia using the facial recognition technology, because we all know that the burden of dementia care has increased substantially due to population aging. The key to the success of dementia care is to early recognize the BPSD and provide appropriate care for those symptoms. The BPSD stands for behavior and psychiatric symptoms of dementia. That includes some positive symptoms like agitation, aggression, and some negative symptoms like depressed or apathy. It has been reported that about one third of the healthcare spendage related to dementia care are spent in the management of BPSD.
So the key of managing the BPSD is dependent on how we early recognize those symptoms and provide necessary individualized nonpharmacological approach to those symptoms, and also trying to reduce the use of anti-psychotic drugs for the long term. And that will be very, very challenging in the long term care setting, because in the long term care setting, usually there will be the non-professional caregivers are taking care of those people with dementia. They need very special training to properly identify BPSD on their own.
So we’re hoping to identify some way to help improve the quality of care in the long term care setting through the modern technology. It has come to our mind that probably we can use the facial recognition technology. It has been reported that through the facial recognition technology, we’ll be able to identify the different moves and potentially the cognitive function of an older person. Then we’re thinking probably we can use this technology to identify the BPSD for those people with dementia in the daycare center. So we conducted our research in the daycare center in the Taipei Veterans General Hospital. That is a relatively small daycare center and more focused on the research and education purpose. The overall capacity of the daycare center is about 30, and about 23 of them participated in this study after the informed consent and the IRB documents.
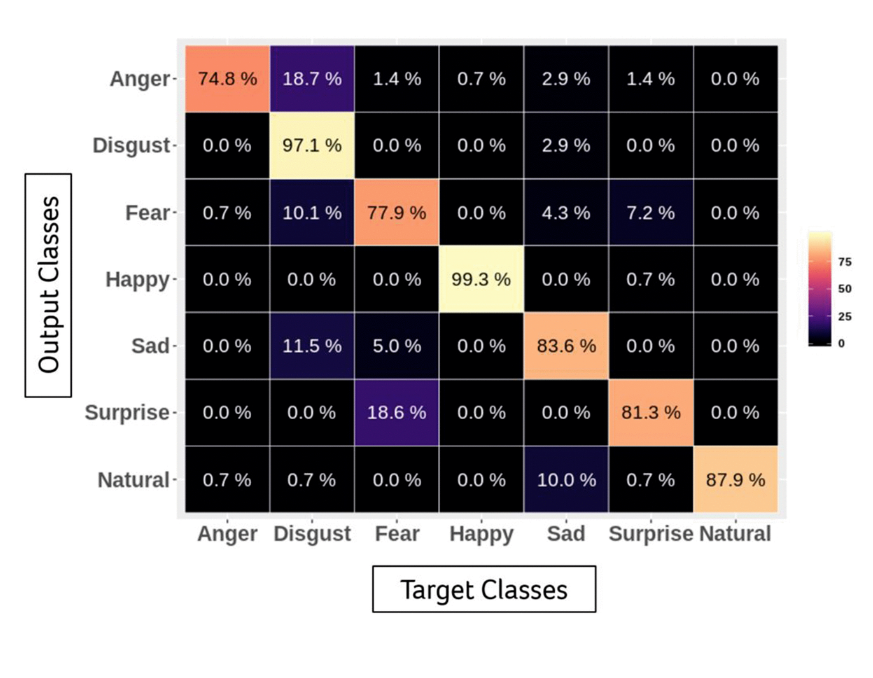
So we decided to use the camera on the ceiling to capture their facial expressions in their daily activities. And we’re thinking whether we can be able to capture their features of their facial expressions so that we can identify the BPSD. You can see that the camera is located on the ceiling at the entrance. We have tried different cameras on the ceilings, but because the older people, they are walking around, sometimes the camera are not able to capture the best quality of the imaging. The camera located at the entrance is probably the best location because it captures their front face every day, so that we can have more images with better quality to generate the prediction model. And then we use this camera to capture all their face, their facial expressions every day when they come to the daycare center.
We have tried several different ways to build our model, because it needs a lot of image data to generate a prediction model, and that we don’t have the size of images. So in the first step we use the open data source using the 100,000 open data source of the faces of different age, sex, and different mood, facial expressions in open dataset, and also using the dataset to generate our prediction model, to predict their seven major facial expressions like sadness, happy, neutrality, or anger. And then we use the publicly available algorithm that has been developed to predict the different moves of through the facial expressions and to validate whether our prediction model is very effective. This confusion metric, you can see that the model we developed is very successful. That is highly compatible with those algorithm developed in the public domains, using a lot of imaging data. So we are quite comfortable with the first algorithm to capture the facial expressions and to differentiate the different mood expression of the older people.
So we move on the second step that we try to collect the data from daycare center and through two, the two stage approach, because we have only limited data sources, data resources. So in the first stage one, we only include six participants of the daycare center and using their image to generate a model based on the previous developed algorithm, predicting the facial expression in different moods. And we use that algorithm to predict the NPI. That is the questionnaire used to define the BPSD. So it is with a total score from zero to 30. And so using the stage one sample, that is a six participant, and to generate a prediction model using machine learning, to predict NPI using the previous algorithm.
Of course we’ve been through the facial extraction and the facial engineering process, and we’ve got a very good prediction result, but because of the limited, sample size, then we’re thinking we should use another sample for the external validation.
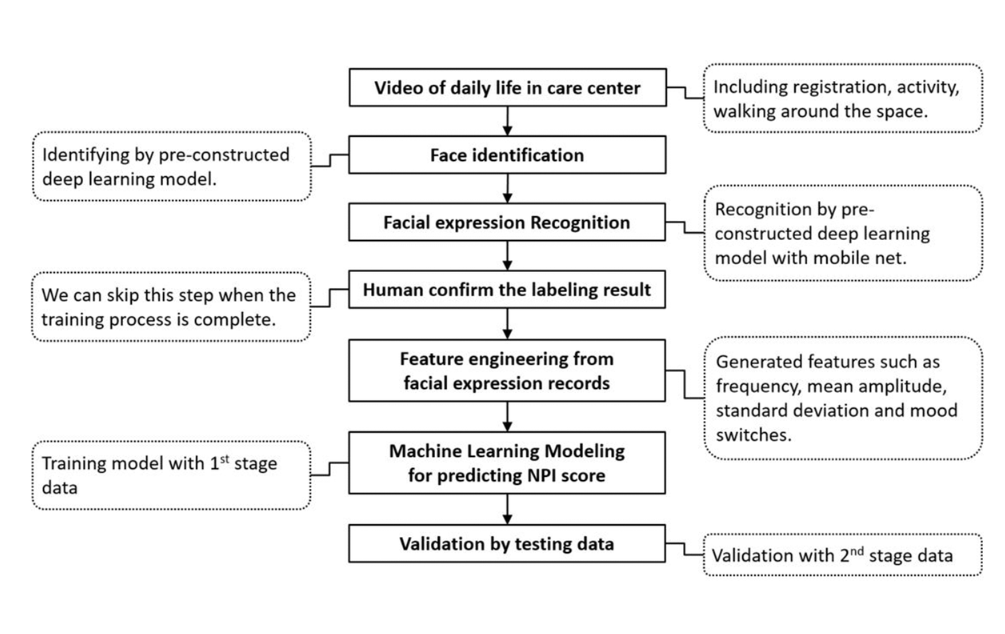
So that moved to the stage two, we are include another 17 subject in the daycare center and trying to develop and validate whether the algorithm really captures the important features are the older persons with dementia and their BPSD. So we did find it is also very good and very well validated algorithm that can successfully identify the BPSD through predicting the NPI scores. And that result would be very important because that is not just a deep learning algorithm. It is a machine learning base in the second part of the study. So that we can try to, again, to capture the feature of their facial expression, whether it is because of sadness or happiness or the amplitude between the mood changes.
So those features will help us to see whether those features are reliable in the clinical practice. And we have done further analysis to see whether we did identify potentially explainable features, and that is very successful. And we did identify each features from the individual mood expression through the transition or the changes between different moods or the amplitude of each mood that is highly correlated with the NPI total score. So it is interesting and important finding and also shows the reliability of our model.
Then we move on to see what’s the main differences between the predicted NPI score and the real assessed NPI score in the daycare center. And overall in the total zero to 30 NPI scores, the predicted differences is only about three scores, something. So it is around 10%. That is pretty satisfactory to help us knowing whether we have successfully identified the people with dementia. They are suffering from BPSD.
So that we can do the individualized care plan to relieve their symptoms and to relieve those may causing all the suffers or the mood distress to all those older people with dementia. And we’re thinking this is a very important approach in the long term care setting to identify BPSD or dementia subject, that we can improve the quality of care in the long-term care setting.
This is our finding, and we’re very glad to let you know that the current work has been successfully become a commercial product that is supported by the Asia company that has becoming a product that can be purchased and used in the daycare center or even using at other long-term care setting. We still improving our algorithm. So hoping that in the future, we’re able to share more research work with you.
This is Dr. Liang-Kung Chen from Taiwan from National Yang Ming Chiao Tung University. Thank you.
Click here to read the full study published by Aging (Aging-US).
AGING (AGING-US) VIDEOS: YouTube | LabTube | Aging-US.com
—
Aging (Aging-US) is an open-access journal that publishes research papers bi-monthly in all fields of aging research and other topics. These papers are available to read at no cost to readers on Aging-us.com. Open-access journals offer information that has the potential to benefit our societies from the inside out and may be shared with friends, neighbors, colleagues, and other researchers, far and wide.
For media inquiries, please contact [email protected].