Dr. Frank Pun, Diana Zagirova, Dr. Anatoly Urban, and Geoffrey Ho Duen Leung from Insilico Medicine Hong Kong Ltd., discuss a research paper they co-authored that was published by Aging (Aging-US) in Volume 15, Issue 18, entitled, “Biomedical generative pre-trained based transformer language model for age-related disease target discovery.”
Behind the Study is a series of transcribed videos from researchers elaborating on their recent studies published by Aging (Aging-US). Visit the Aging (Aging-US) YouTube channel for more insights from outstanding authors.
—
Good day, everyone. I’m Frank Pun, Head of Insilico Medicine, Hong Kong office where we focus on AI-driven target discovery. Recently we published a paper in Aging, titled “Biomedical generative pre-trained based transformer language model for age-related disease target discovery.”
With me today, our first author of the paper, Diana. Can you briefly introduce your BioGPT paper on what is the significance of this study? Thank you.
Sure. We just published a paper in Aging journal and it’s related on the usage of LLM, so large language models, on the target discovery. Large language models already have shown that they have amazing opportunities for solving different tasks, but still there is a challenge of efficiently extracting information from them.
So we tried to address this challenge and we developed an approach: How we could extract the information from large language models and apply this information for therapeutic target discovery, specifically in aging. So during (during) our work we actually discovered several unique properties of LLMs that could be useful for first AI research and one of them that – even if you have a demand specific large language model – it was to train with additional information that is related for your task. So for example, we used BioGPT. This is a language model developed by Microsoft and it was trained on PubMed texts that already biology specific. But we have a really unique task, we need to have to discover new therapeutics.
So we decided to train our model with additional corpus of texts from National Institute of Health to have this task-specific text. And in our validation, we have shown that performance of BioGPT has been boosted. Certainly the importance of our work is its results because we actually focused on identification of dual effect targets, that could both fight aging and intervene in 14 age-related diseases and we found 2 among other results. We also found 2 potential anti-aging targets that were not previously described. So we showed that large language models have an opportunity to extract novel information when the problem of information extracted is tackled properly.
I was deeply impressed the first time I saw the results. It is very amazing. Can I ask how did you come up with this idea and what is the rationale behind the model?
Yeah, sure. So the reason we decided to use a large language model for target discovery is because it has shown to be quite effective in recognizing intricate patterns inside of the text. So the goal when we identify the novel targets is pretty similar. We want to have to identify the connection between the protein and the disease. So we decided to test the hypothesis that LLMs could help us in this task.
Especially it’s important, I think in aging research, because aging process is multi-faced and have multiple players within it. The approach is based on BioGPT that we enhanced with additional text and then the approach itself is pretty straightforward.
We first give BioGPT an unfinished sentence or prompt that’s related to the aging. For example, “it was the gene that’s related to aging is…” and then asked LLM to finish this prompt. But instead of extracting only a name of 1 gene, we actually extracted the probabilities of each gene to be in this position, to be the last word of this sentence. This way we’re able to rank all the genes and extract the top ones as a possible targets. So this approach is really flexible and actually it could be used to different applications when the ranking is needed.
I agree with you. So hopefully we can apply the same model, of course with some modifications to do other biomedical research as well. So the next question is to Anatoly. While we know that earlier this year you also published a paper, Precious1GPT on aging study. It is also very cool paper, so compared to the Precious1GPT, what do you think about GPT’s performance in aging research and which one do you like more? Could we combine the 2 together to facilitate the aging research as well?
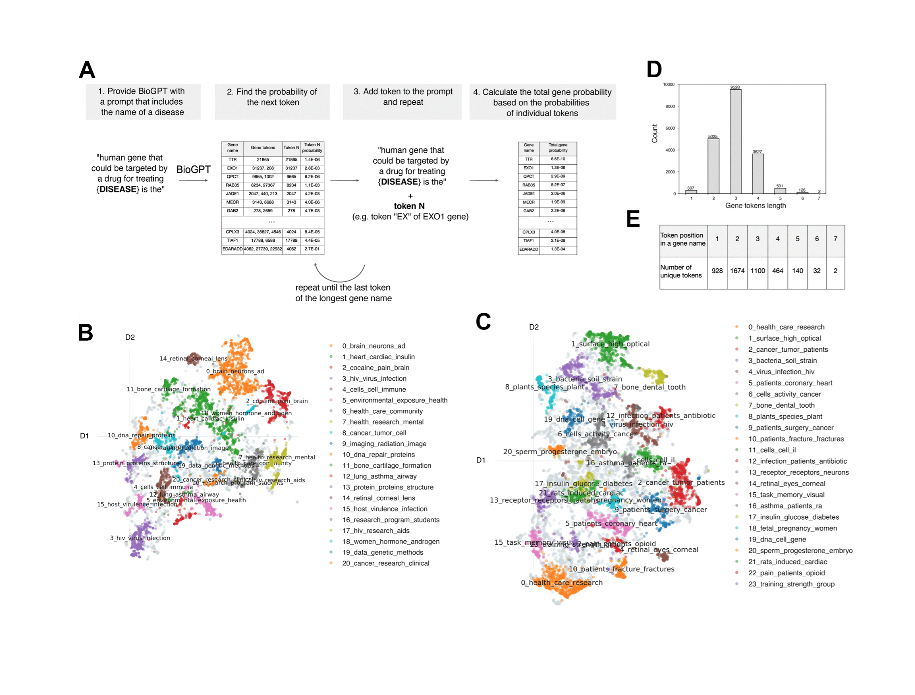
These models are quite different but complementary in their approach. Precious1GPT excels in analyzing raw biological data such as expression and methylation, and uses this knowledge to predict disease status. However, it’s limited to the experimental PandaOmics data we provide. In contrast, BioGPT relies on text data, mainly research papers and generalized information. It processes data from papers and leverages its large language model capabilities to assist the researchers. Together, they cover different aspects of aging research and compliment each other in their respective leads.
I appreciate both models equally. We have powerful tools that can help our researchers navigate the vast amounts of data and character in modern research, both in textual and Omics form. Each one has its unique strengths and contributes to understanding of and age-related diseases. By combining strengths of Precious1GPT and BioGPT, for example, we can generate new hypothesis using Omics data and then consult BioGPT to find supporting evidence in the existing scientific literature. The synergy can accelerate the discovery process and lead to breakthroughs in aging research.
Thank you Anatoly. And it’s great to hear that we can combine the 2 to have the synergy effects. So Geoffrey, the next question is to you. So we published some papers on the AI driven target discovery, especially for the dual purpose targets for aging research as well. So how does the current study add value and relates to our previous works?
The combination of multiple models, including Omics data and text based data, to identify targets in age associated diseases and aging. This model used the dual purpose targets from a different perspective, that is complicity driven by the last language model and without the use of modulus data. So in this case, we don’t have to sedate the dataset ourselves. And by comparing the 2 sets of targets, prioritized by both methods, we may find some overlaps of the targets to support our model and our prediction. What will be interesting is when we find some targets that interact with each other. So in this case, we may find an explored molecular mechanisms and underlying aging and age associated diseases. So in that case, we’ll improve the research in aging.
Thank you, Geoffrey. So what will be the future directions? Diana, may I have your comment first?
I think the future direction for our search will be extending the scope of application of AI because improvements of AI showed that these models could be applied to a variety of tasks specifically in the biomedical field. So I think the possibility for AI is truly fascinating.
I think future directions include improving models by incorporating more complex and powerful architectures and integrate in additional data modalities. As we progress towards models, we have deeper understanding of biological system. We’ll definitely uncover in the applications for generating AI. Eventually, we may reach a point where we can simply ask a model to modify a biological system in desired direction or just create a cure for specific disease and receive the answer in plain text.
In aging research particularly, I think it may be interesting and promising to use for identifying some repurposing drug candidates, so possibly they are FDA approved. We may use this model to prioritize or to screen for the compounds that could potentially be related to aging and other age associated diseases. Then we can further investigate and study those compounds or drugs.
I’m sure it is clear that our collective efforts are advancing the AI driven target discovery for aging research and let’s continue to collaborate and drive the innovation in healthcare.
Click here to read the full study published by Aging (Aging-US).
—
Aging (Aging-US) is an open-access journal that publishes research papers bi-monthly in all fields of aging research and other topics. These papers are available to read at no cost to readers on Aging-us.com. Open-access journals offer information that has the potential to benefit our societies from the inside out and may be shared with friends, neighbors, colleagues, and other researchers, far and wide.
For media inquiries, please contact [email protected].